Predictive Maintenance (PdM) Market Status and Trend Report (2023-2032)
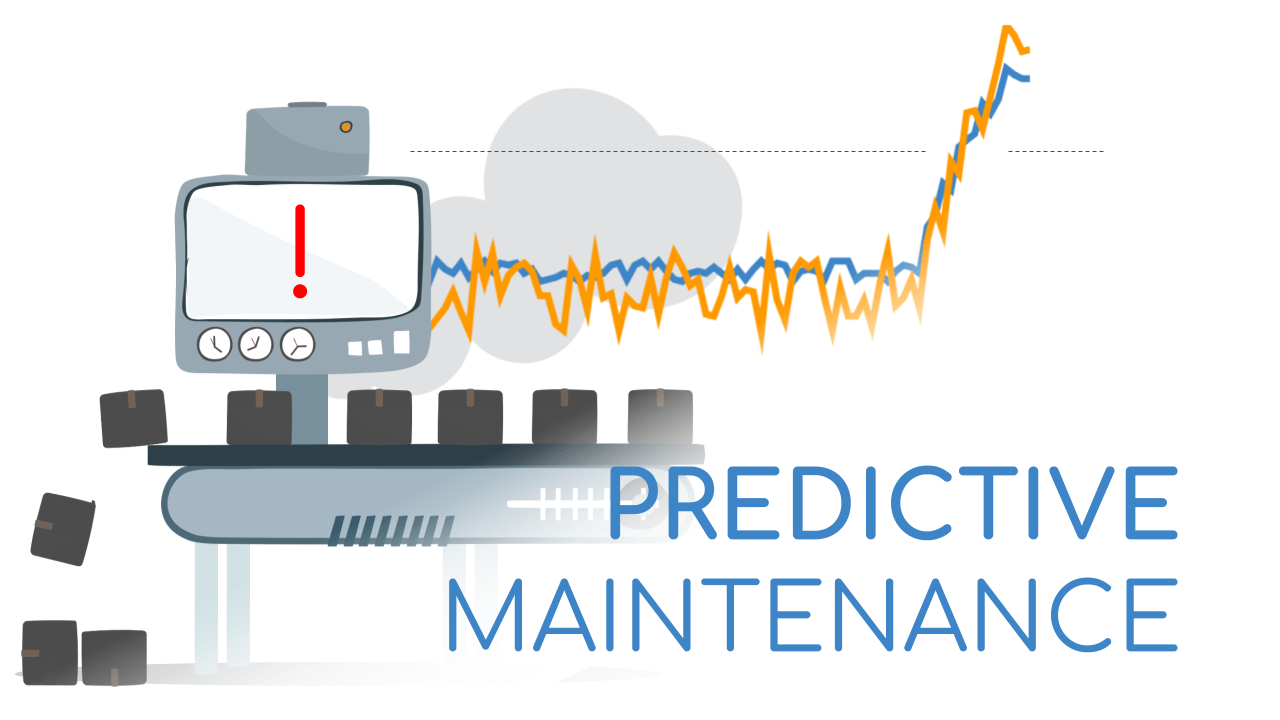
In today's fast-paced and highly competitive business landscape, companies are continuously seeking innovative ways to optimize their operations and reduce costs. One approach that has gained significant traction is predictive maintenance (PdM). By harnessing the power of data, predictive maintenance enables businesses to move from reactive to proactive maintenance strategies, ensuring better equipment performance, increased uptime, and improved overall efficiency. The Predictive Maintenance (PdM) market growth is projected to grow from USD21.83 Billion in 2022 to USD 111.30 billion by 2030, exhibiting a compound annual growth rate (CAGR) of 26.20% during the forecast period (2022 - 2030).
Introduction:
Predictive maintenance refers to the practice of using advanced analytics techniques, such as machine learning and artificial intelligence, to predict equipment failures and perform maintenance activities proactively. Rather than relying on scheduled or reactive maintenance approaches, PdM leverages real-time data from sensors, Internet of Things (IoT) devices, and other sources to identify patterns, detect anomalies, and forecast potential failures. This data-driven approach helps businesses optimize maintenance schedules, reduce downtime, and avoid costly repairs.
Request a Free PDF Sample Report
Benefits of Predictive Maintenance:
1) Cost Reduction
One of the primary advantages of implementing predictive maintenance is its potential for cost reduction. By detecting potential failures before they occur, businesses can avoid costly breakdowns and unplanned downtime. Proactive maintenance enables organizations to plan maintenance activities effectively, ensuring that resources are allocated efficiently and minimizing disruptions to operations. Additionally, predictive maintenance helps optimize inventory management by ensuring that spare parts and components are available when needed, reducing the need for excessive inventory and associated carrying costs.
2) Increased Equipment Uptime
Predictive maintenance plays a crucial role in maximizing equipment uptime. By continuously monitoring equipment performance and identifying early signs of degradation or malfunctions, businesses can address issues before they escalate into significant problems. This proactive approach helps minimize unplanned downtime, improve overall equipment effectiveness (OEE), and ensure seamless production processes.
3) Improved Safety
Another key benefit of predictive maintenance is its contribution to enhancing safety in industrial settings. By regularly monitoring equipment condition and performance, businesses can identify potential safety risks and take preventive measures. Timely maintenance reduces the likelihood of accidents caused by faulty equipment, protecting both employees and assets. Predictive maintenance also helps organizations comply with safety regulations and standards, avoiding potential penalties and legal consequences.
4) Enhanced Productivity
Predictive maintenance drives productivity improvements by optimizing maintenance activities and minimizing disruptions. By preventing unexpected equipment failures, businesses can maintain smooth production workflows and meet customer demands without delays. Additionally, predictive maintenance enables organizations to schedule maintenance during planned downtimes, minimizing the impact on productivity. This proactive approach reduces the need for emergency repairs, allowing maintenance teams to focus on value-added tasks rather than firefighting issues.
Key Technologies in Predictive Maintenance:
Predictive maintenance relies on several key technologies that enable effective data analysis and predictive modeling.
1) Machine Learning and Artificial Intelligence
Machine learning algorithms and artificial intelligence play a crucial role in predictive maintenance. These technologies analyze historical and real-time data to identify patterns, anomalies, and correlations that can indicate potential failures. By continuously learning from new data, machine learning models become increasingly accurate in predicting equipment conditions and performance. Artificial intelligence techniques, such as neural networks, decision trees, and deep learning, enhance the predictive capabilities and enable proactive maintenance decision-making.
2) Internet of Things (IoT)
The Internet of Things (IoT) is a fundamental component of predictive maintenance. IoT devices and sensors collect vast amounts of data from equipment, including temperature, vibration, pressure, and other relevant parameters. This real-time data is transmitted to a central analytics platform, where it is processed and analyzed. The IoT enables remote monitoring and condition-based maintenance, providing valuable insights for proactive maintenance strategies.
3) Data Analytics and Predictive Modeling
Data analytics and predictive modeling techniques are essential for extracting meaningful insights from the collected data. Advanced analytics tools, such as statistical analysis, data mining, and predictive modeling algorithms, enable businesses to uncover hidden patterns, detect anomalies, and forecast potential failures. These insights help optimize maintenance schedules, predict equipment lifecycles, and determine the most effective maintenance strategies.
Implementation of Predictive Maintenance:
Implementing predictive maintenance requires careful planning and execution. The following steps outline the key considerations in deploying a successful PdM strategy.
1) Data Collection and Storage
To implement predictive maintenance, businesses need to collect and store relevant data from equipment and sensors. This includes real-time sensor data, historical maintenance records, and other contextual information. Robust data collection mechanisms and secure storage infrastructure are necessary to ensure data integrity and accessibility.
2) Sensor Deployment and Monitoring
Strategic sensor deployment is crucial for effective predictive maintenance. Sensors should be strategically placed to capture essential equipment parameters and performance indicators. These sensors continuously monitor equipment conditions and transmit real-time data to the central analytics platform. Regular sensor calibration and maintenance are necessary to ensure accurate data collection.
3) Predictive Analytics Algorithms
Sophisticated predictive analytics algorithms are the backbone of successful PdM implementations. These algorithms analyze collected data, identify patterns, and detect anomalies or early signs of equipment degradation. Predictive models can generate alerts, notifications, or work orders based on the identified patterns, enabling proactive maintenance interventions.
4) Maintenance Scheduling and Optimization
Based on the insights provided by predictive maintenance, businesses can optimize their maintenance schedules. Proactive maintenance activities can be scheduled during planned downtimes, minimizing disruptions to operations. Predictive maintenance insights also help prioritize maintenance tasks based on equipment criticality, availability of resources, and potential impact on production processes.
Industries Adopting Predictive Maintenance:
Predictive maintenance finds applications across various industries, enabling businesses to optimize their maintenance practices and improve operational efficiency.
1) Manufacturing
Manufacturing industries extensively use predictive maintenance to reduce unplanned downtime, optimize maintenance costs, and enhance equipment performance. Proactive maintenance strategies help manufacturers meet production targets, minimize scrap rates, and improve product quality.
2) Energy and Utilities
In the energy and utilities sector, predictive maintenance plays a crucial role in optimizing the performance of power plants, oil refineries, and renewable energy installations. By detecting potential failures in advance, energy companies can minimize costly disruptions and ensure reliable energy supply.
3) Transportation
The transportation industry benefits from predictive maintenance by optimizing the maintenance of vehicles, aircraft, and railway systems. Proactive maintenance helps prevent breakdowns and accidents, ensuring passenger safety and improving the overall reliability of transportation networks.
4) Healthcare
Predictive maintenance is also gaining traction in the healthcare sector, where it supports the maintenance of critical medical equipment and infrastructure. Proactive maintenance ensures that medical facilities operate smoothly, minimizing downtime and providing timely healthcare services.
5) Aerospace and Defense
The aerospace and defense industry relies on predictive maintenance to ensure the optimal performance and safety of aircraft, defense systems, and satellite networks. Predictive maintenance helps identify potential equipment failures and ensures the availability of mission-critical assets.
Challenges in Implementing Predictive Maintenance:
While predictive maintenance offers numerous benefits, several challenges must be addressed for successful implementation.
1) Data Quality and Availability
The success of predictive maintenance heavily relies on the quality and availability of data. Businesses need access to accurate and timely data from equipment sensors and other relevant sources. Data cleansing, validation, and integration processes are necessary to ensure the reliability of predictive maintenance insights.
2) Skill Gap and Workforce Training
Implementing predictive maintenance requires skilled personnel who can analyze data, develop predictive models, and interpret insights. Organizations may face a skill gap in data analytics and machine learning. Workforce training programs and partnerships with data science experts can bridge this gap and enable successful implementation.
3) Cost of Implementation
Implementing predictive maintenance systems and infrastructure can involve significant upfront costs. This includes investment in sensors, data storage infrastructure, analytics platforms, and skilled personnel. Organizations need to carefully evaluate the return on investment (ROI) and long-term cost savings associated with predictive maintenance.
4) Integration with Existing Systems
Integrating predictive maintenance solutions with existing systems, such as enterprise resource planning (ERP) or computerized maintenance management systems (CMMS), can be challenging. Seamless data flow, interoperability, and compatibility between systems are essential for successful implementation.
Future Trends in Predictive Maintenance:
Predictive maintenance is a rapidly evolving field, and several trends are shaping its future.
1) Edge Computing and Real-time Analytics
Edge computing, where data processing occurs closer to the data source, is gaining prominence in predictive maintenance. Real-time analytics at the edge enable faster insights, quicker response times, and reduced reliance on cloud infrastructure. This trend enables proactive maintenance decisions in near real-time, ensuring optimal equipment performance.
2) Predictive Maintenance as a Service
Predictive maintenance as a service (PdMaaS) models are emerging, where businesses can outsource their predictive maintenance needs to specialized providers. PdMaaS offers scalable and cost-effective solutions, particularly for smaller organizations without extensive data analytics capabilities.
3) Integration with Augmented Reality and Virtual Reality
Augmented reality (AR) and virtual reality (VR) technologies are increasingly integrated with predictive maintenance workflows. AR and VR provide maintenance technicians with interactive, visual guidance, enabling them to perform tasks more efficiently and accurately. This integration improves maintenance effectiveness and reduces human error.
4) Predictive Maintenance for Autonomous Vehicles
As autonomous vehicles become more prevalent, predictive maintenance plays a critical role in ensuring their safe and efficient operation. Predictive maintenance insights help detect potential failures in autonomous vehicle systems, enabling timely maintenance interventions and preventing accidents.
Conclusion:
Predictive maintenance is revolutionizing the way businesses approach equipment maintenance. By leveraging data analytics, machine learning, and IoT technologies, organizations can move from reactive to proactive maintenance strategies. Predictive maintenance offers cost reduction, increased equipment uptime, improved safety, and enhanced productivity across various industries. However, challenges related to data quality, skill gaps, implementation costs, and system integration must be addressed for successful implementation. As predictive maintenance continues to evolve, future trends such as edge computing, PdMaaS, AR/VR integration, and maintenance for autonomous vehicles will shape the industry.