Global Deep Learning Market Analysis: Opportunities and Challenges Ahead
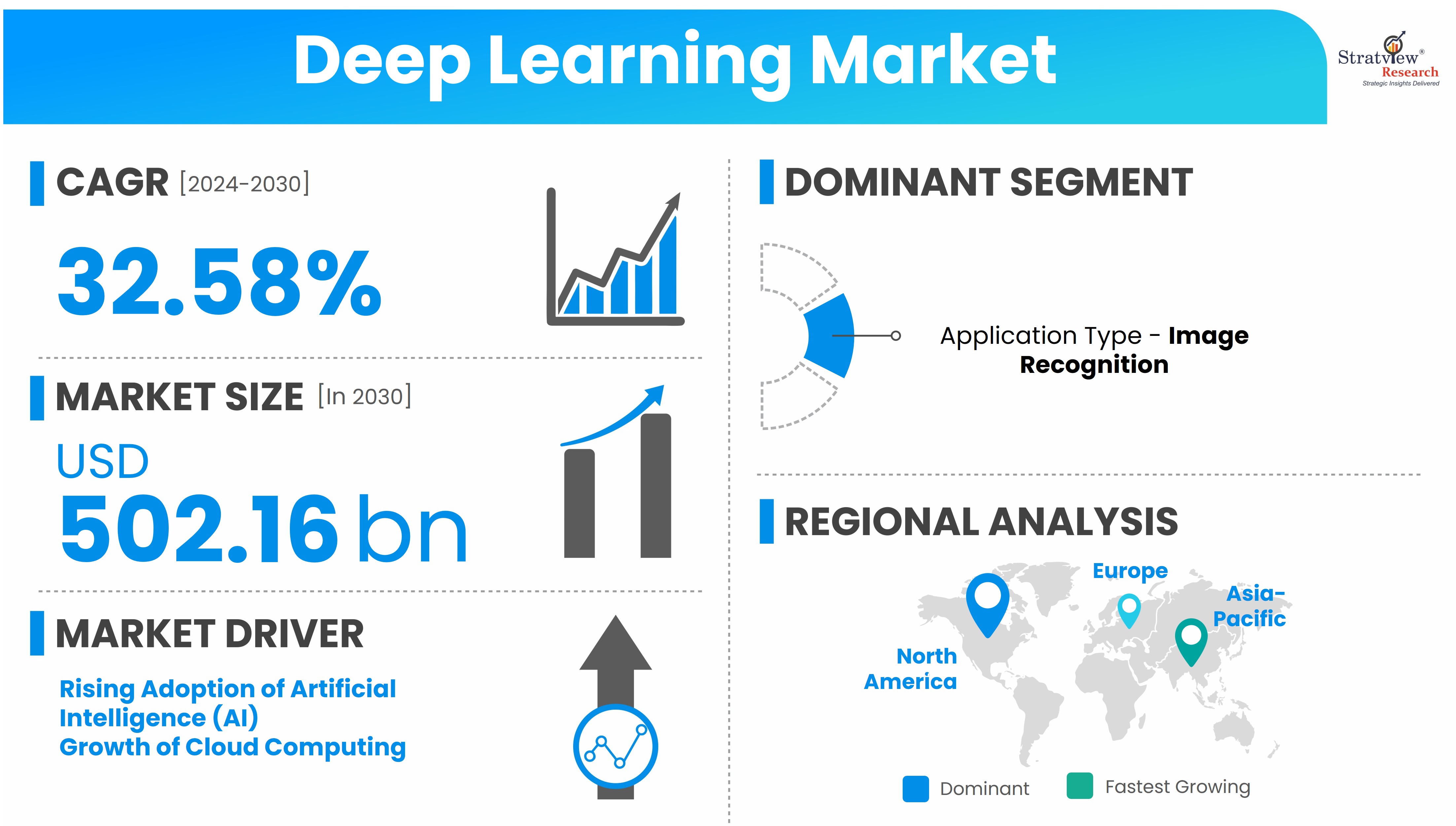
The global deep learning market has witnessed remarkable growth over the past few years, driven by advancements in artificial intelligence (AI), big data, and computing power. This expansion presents a wealth of opportunities across various industries, but also poses unique challenges that need to be addressed for sustainable development. Here, we analyze the opportunities and challenges shaping the deep learning market’s future.
According to Stratview Research, the deep learning market was estimated at USD 69 billion in 2023 and is likely to grow at a CAGR of 32.58% during 2024-2030 to reach USD 502.16 billion in 2030.
Opportunities Driving the Deep Learning Market
- Widespread Industry Adoption: Deep learning’s applications are vast, ranging from healthcare and automotive to finance and retail. In healthcare, deep learning is enhancing medical image analysis, improving diagnostics, and accelerating drug discovery. In automotive, it’s enabling autonomous vehicles and enhancing safety features through real-time object detection. As businesses across these industries look to leverage AI for automation and efficiency, deep learning offers the tools to drive these innovations.
- Big Data and Data Analytics: The explosion of data from IoT devices, social media, and enterprise systems provides an invaluable resource for training deep learning models. With its ability to process and analyze vast datasets, deep learning technology enables organizations to extract actionable insights, optimize operations, and improve customer experiences. The increasing availability of big data across sectors like retail, manufacturing, and energy further accelerates the demand for deep learning solutions.
- Cloud Computing Integration: The growth of cloud computing has lowered the barriers to entry for businesses seeking to implement deep learning. Cloud service providers like AWS, Google Cloud, and Microsoft Azure now offer scalable infrastructure that supports deep learning development, allowing companies of all sizes to access AI tools and deploy machine learning models without significant upfront investment. This democratization of AI helps fuel market expansion.
- Technological Advancements in Hardware and Algorithms: Continued innovations in hardware, such as specialized AI chips (e.g., GPUs, TPUs), have drastically improved the computational efficiency of deep learning models. In parallel, advances in algorithms, such as transformers for natural language processing (NLP) and GANs for creative AI applications, are further pushing the boundaries of what deep learning can achieve.
Challenges Facing the Deep Learning Market
- Data Privacy and Security Concerns: The massive data requirements for deep learning models raise significant privacy and security concerns. As sensitive data is processed, organizations must navigate stringent data protection regulations, such as GDPR, to ensure compliance and build consumer trust. Balancing data utilization with security remains a key challenge.
- High Computational Costs: Training deep learning models demands substantial computational resources, which can be costly for organizations. Although cloud-based solutions offer more accessible pricing, the energy consumption and associated environmental impact of training large AI models are growing concerns that the industry must address.
- Lack of Skilled Workforce: The deep learning market is still facing a shortage of highly skilled professionals capable of developing and deploying advanced AI models. As demand for deep learning solutions grows, companies must invest in talent acquisition, training, and education to ensure a steady pipeline of expertise.
- Model Interpretability and Bias: Deep learning models, especially deep neural networks, are often regarded as “black boxes” due to their complexity. The lack of transparency in how models make decisions can be problematic in high-stakes fields like healthcare and finance. Additionally, biases in training data can lead to skewed outcomes, raising ethical concerns.
Conclusion
The deep learning market holds immense potential, driven by increasing adoption across industries, big data proliferation, and technological innovations in hardware and algorithms. However, challenges such as data privacy, high computational costs, and workforce shortages must be tackled to unlock its full capabilities. As these issues are addressed, the market is poised for continued growth, offering transformative solutions to businesses and industries worldwide.